

Economics and Business
Quarterly Reviews
ISSN 2775-9237 (Online)







Published: 26 July 2021
Modelling & Forecasting Volatility of Daily Stock Returns Using GARCH Models: Evidence from Dhaka Stock Exchange
Md. Tuhin Ahmed, Nurun Naher
South Asian Network on Economic Modeling (Bangladesh), Auburn University (United States)
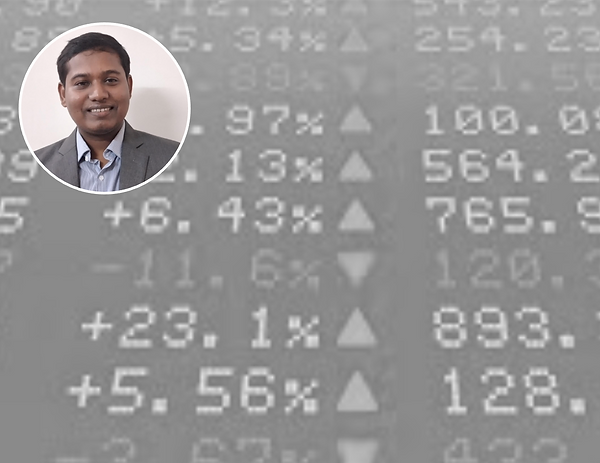
Download Full-Text Pdf

10.31014/aior.1992.04.03.371
Pages: 74-89
Keywords: ARCH, GARCH, Volatility, Forecasting, DSE
Abstract
Modelling volatility has become increasingly important in recent times for its diverse implications. The main purpose of this paper is to examine the performance of volatility modelling using different models and their forecasting accuracy for the returns of Dhaka Stock Exchange (DSE) under different error distribution assumptions. Using the daily closing price of DSE from the period 27 January 2013 to 06 November 2017, this analysis has been done using Generalized Autoregressive Conditional Heteroscedastic (GARCH), Asymmetric Power Autoregressive Conditional Heteroscedastic (APARCH), Exponential Generalized Autoregressive Conditional Heteroscedastic (EGARCH), Threshold Generalized Autoregressive Conditional Heteroscedastic (TGARCH) and Integrated Generalized Autoregressive Conditional Heteroscedastic (IGARCH) models under both normal and student’s t error distribution. The study finds that ARMA (1,1)- TGARCH (1,1) is the most appropriate model for in-sample estimation accuracy under student’s t error distribution. The asymmetric effect captured by the parameter of ARMA (1,1) with TGARCH (1,1), APARCH (1,1) and EGARCH (1,1) models shows that negative shocks or bad news create more volatility than positive shocks or good news. The study also provides evidence that student’s t distribution for errors improves forecasting accuracy. With such an error distribution assumption, ARMA (1,1)-IGARCH (1,1) is considered the best for out-of-sample volatility forecasting.
References
Abd El Aal M. A. (2011). Modelling and forecasting time varying stock return volatility in the Egyptian stock market. International Research Journal of Finance and Economics, 78, 96-113.
Abdullah, S. M., Siddiqua, S., Siddiquee, M. S. H., & Hossain, N. (2017). Modelling and forecasting exchange rate volatility in Bangladesh using GARCH models: A comparison based on normal and student’s t-error distribution. Financial Innovation, 3 (18).
Andersen, T. G., & Bollerslev, T. (1998). Answering the Skeptics: yes, standard volatility models do Provide accurate forecasts. International Economic Review,39(4), 885-905
Banumathy, K., & Azhagaiah, R. (2013). Modelling Stock Market Volatility: Evidence from India. Managing Global Transitions, University of Primorska, Faculty of Management Koper, 13(1),27-41.
Bollarslev, T. (1986). Generalized autoregressive conditional heteroscedasticity. Journal of Econometrics, 31 (3), 307-327.
Brooks, C. (2002).Introductory Econometrics for Finance. Cambridge University Press.
Dickey, D. A., & Fuller, W. A. (1979). Distribution of the estimators for autoregressive time series with a unit root. Journal of the American Statistical Association,74 (366), 427-431.
Ding, Z., Granger, C.W. J., & Engle, R. F. (1993). Long memory properties of stock market returns and a new model. Journal of Empirical Finance, 1(1), 83-106.
Engle, R.F. (1982). Autoregressive conditional heteroscedasticity with estimates of the variance of UK inflation. Econometrica. 50(4), 987-1007.
Floros, C. (2008). Modelling Volatility Using GARCH Models: Evidence from Egypt and Israel. Middle Eastern Finance and Econometrics, 2, 31-41.
Glosten, L. R., Jagannathan, R., & Runkle, D. E. (1993). On the relation between the expected value and the volatility of the nominal excess return on stocks. The Journal of Finance, 48(5), 1779-1801.
Huq, M. M., Rahman, M. M., Rahman, M. S., Shahin, M. A., & Ali, M. A. (2013). Analysis of volatility and forecasting general index of Dhaka stock exchange.”American Journal of Economics,3(5), 229-242.
Karmakar, M. (2005). Modelling conditional volatility of the Indian stock markets. Vikalpa, 30(3): 21-38
Mandelbrot B. (1963). The variation of certain speculative prices. The Journal of Business, 36(4), 394-419.
models and Student-t densities: Evidence from Chittagong Stock Exchange. The International Journal of Business and Finance Research, 3, 19-34.
Nelson, D. B. (1991). Conditional Heteroscedasticity in Asset Returns: a new approach. Econometrica, 59, 347-370.
Niyitegeka O., & Tewari, D. D. (2013). Volatility Clustering of the Johannesburg Stock Exchange: Investigation and analysis. Mediterranean Journal of Social Science,4(14), 621-626.
Parvaresh M., & Bavaghar M. (2012). Forecasting volatility in Tehran stock market with GARCH models. Journal of Basic and Applied Scientific Research, 2(1), 150-155
Peters J. (2001). Estimating and forecasting volatility of stock indices using asymmetric GARCH models and (skewed) student’s t densities.” International Journal of Business Management, 161-176
Qamruzzaman, M. (2015). Estimating and forecasting volatility of stock indices using asymmetric GARCH models and Student-t densities: Evidence from Chittagong Stock Exchange. International Journal of Business and Finance Management Resaerch, 2(2), 19-34.
Zakaria S. S. A., & Winker, P. (2012). Modelling Stock Market Volatility using Univariate GARCH Models: Evidence from Sudan and Egypt. International Journal of Economics and Finance, 4(8),161-76.
Zakoian, J. (1994). Threshold heteroscedasticity models. Journal of Economic Dynamics and Control, 18(5), 931-955.